Science cluster

Summary
The AI-SCOPE project engages the European photon and neutron (PaN) science community to enhance the frequency and quality of FAIR-compliant data submissions to EOSC-indexed databases, such as the ESRF data portal or Zenodo.
The project stands to dramatically enhance data FAIRness and beamtime efficiency, by introducing a live AI analysis tool for surface scattering experiments. By rigorously applying it to a diverse array of pre-existing scattering data and enabling live analysis of data from a multitude of sample types we will create a well-curated dataset with detailed annotations in EOSC indexed databases, and lay the groundwork for more uploads with metadata in the future. Both the AI-tool and the curated database will unlock new possibilities for data reuse and collaborative scientific exploration of X-ray and neutron data with machine learning helping also AI-guided materials discovery.
Challenge
Open Science Service, Main RI concerned, Cross-domain/Cross-RI
The main challenge of the AI-SCOPE project is to increase the frequency and quality of FAIR-compliant data submissions to EOSC-indexed databases, such as ESRF data portal or Zenodo, by addressing the current lack of well-annotated surface scattering data. Researchers often neglect valuable data due to time constraints, selective attention, and lack of incentives for annotating and sharing datasets. The project seeks to address these issues by introducing an AI tool that enhances real-time data analysis during beamtime and automates the generation of rich metadata, encouraging the reuse and FAIR submission of data in open databases. It must overcome the difficulties of reusing raw data by integrating standardised metadata and facilitating searches for specific materials and structures.
Solution
The project tri-national team will introduce a sophisticated AI analysis tool for surface scattering experiments that performs an automated initial analysis, and simultaneously generates rich metadata annotations. This dual capability incentivises researchers to utilise the tool, as it streamlines their workflow and gives “live” feedback during experimental sessions at large scale facilities (“beamtimes”).
The AI-tool will be designed to use AI to estimate structural sample parameters (such as film thickness, material densities, contact planes and unit cell dimensions) and feed these into the database using standardised metadata formats. This feature ensures the database can be searched for specific materials and structures from the automated AI analysis, thereby avoiding the pitfalls of a hard to re-use raw data dump and increasing reusability within the scientific ecosystem of EOSC / PaNOSC.
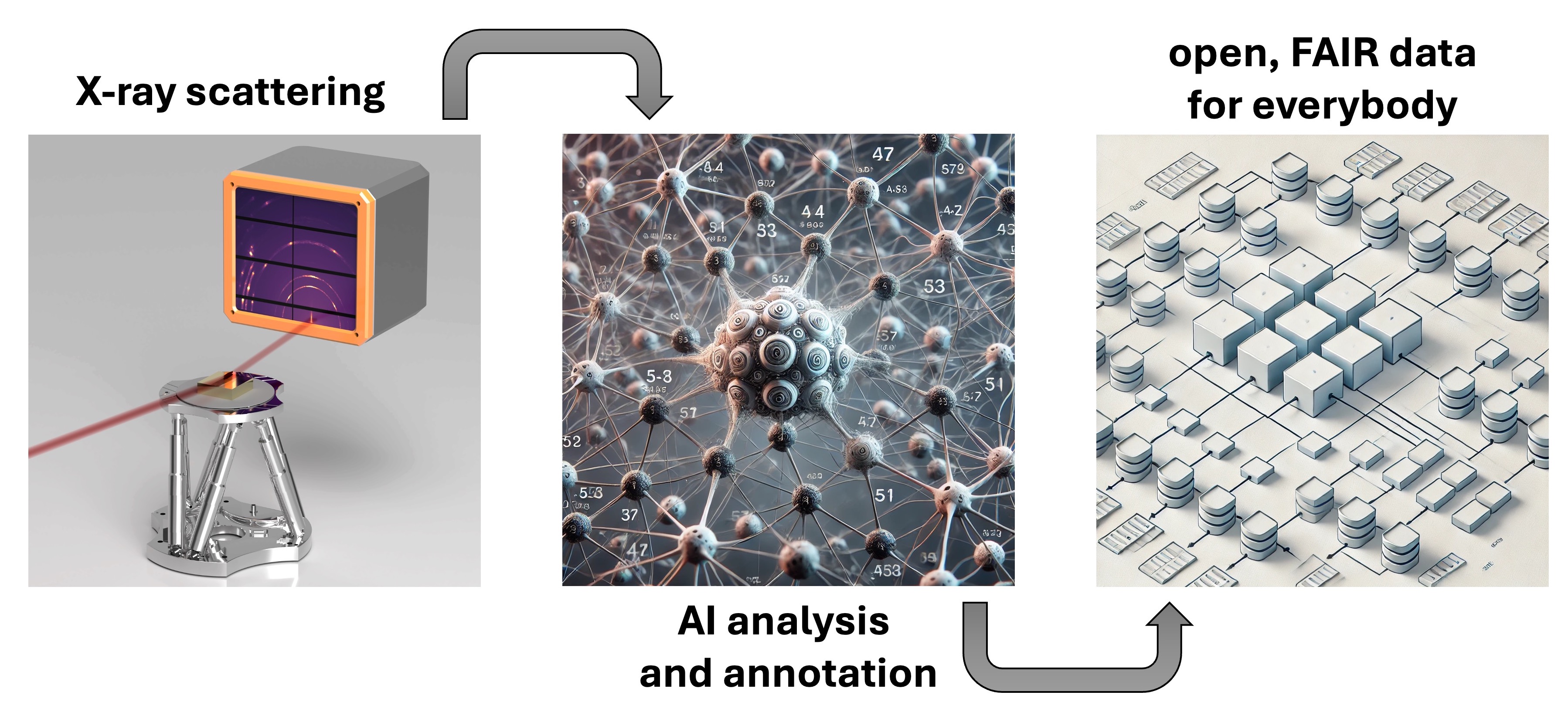
Scientific Impact
The integration of AI-driven analysis tools to EOSC services and the creation of a curated FAIR database represent pivotal advancements in the field of surface scattering. Building on the state-of-the-art, the project will develop individual solutions and make them available, both at large-scale facilities and online, through EOSC services.
By automating the analysis processes and enriching datasets with preliminary insights, these innovations enable a more comprehensive exploration of experimental data. This approach not only accelerates the discovery of novel materials but also facilitates meta-studies and machine learning applications in materials discovery. Moreover, providing a curated database as a nucleus and incentivising community contributions through accessible software tools, promises to result in a significantly higher user uptake of EOSC services and open databases, such as Zenodo. Similar to classical machine learning datasets that get used as benchmarks in the respective communities (e.g., ImageNet competition), the project strives to provide such a benchmark dataset to the European community (and beyond) to start also the comparison of different machine learning algorithms on real-world datasets.
The project thereby serves as a catalyst for filling Zenodo and EOSC nodes (e.g., ESRF data portal) with valuable, actionable data, thus propelling the surface scattering field toward unprecedented collaborative and scientific achievements.
Results to date
Test
Test
Principal investigator
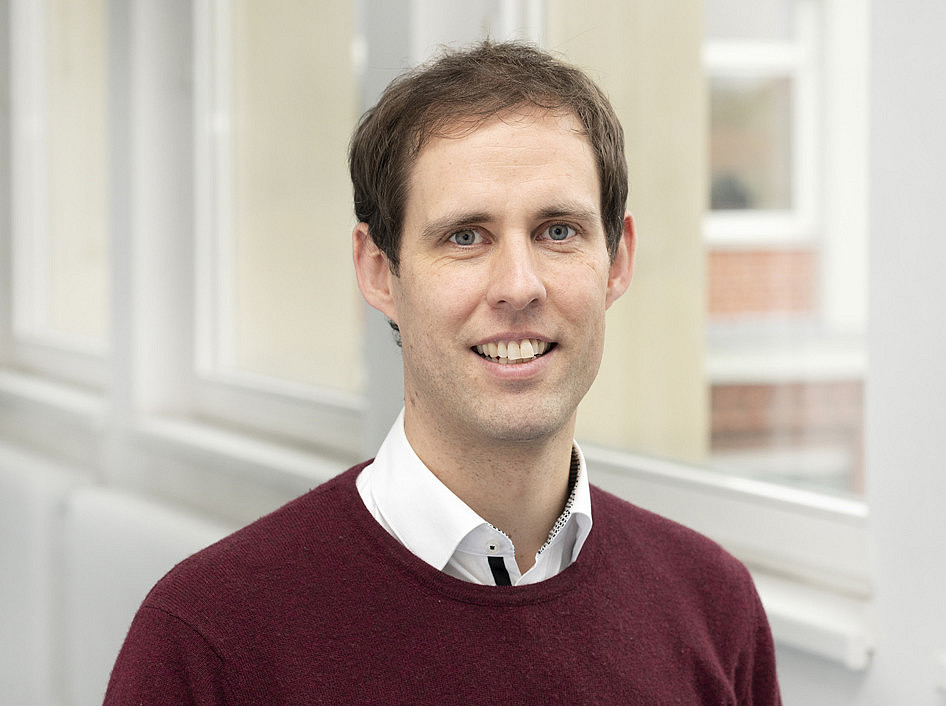
After studying physics at the Ludwig Maximilian University in Munich, Stefan Kowarik completed his doctorate in physical chemistry at Oxford in 2006. Post-doctoral stays took him to the universities of Tübingen and Cornell, and an Alexander von Humboldt Fellowship took him to Berkeley in California. In 2009, Humboldt University in Berlin appointed him to a junior professorship in physics. From 2017, he worked at the Federal Institute for Materials Research and Testing. Since 2020, he has been working in physical chemistry at the University of Graz.
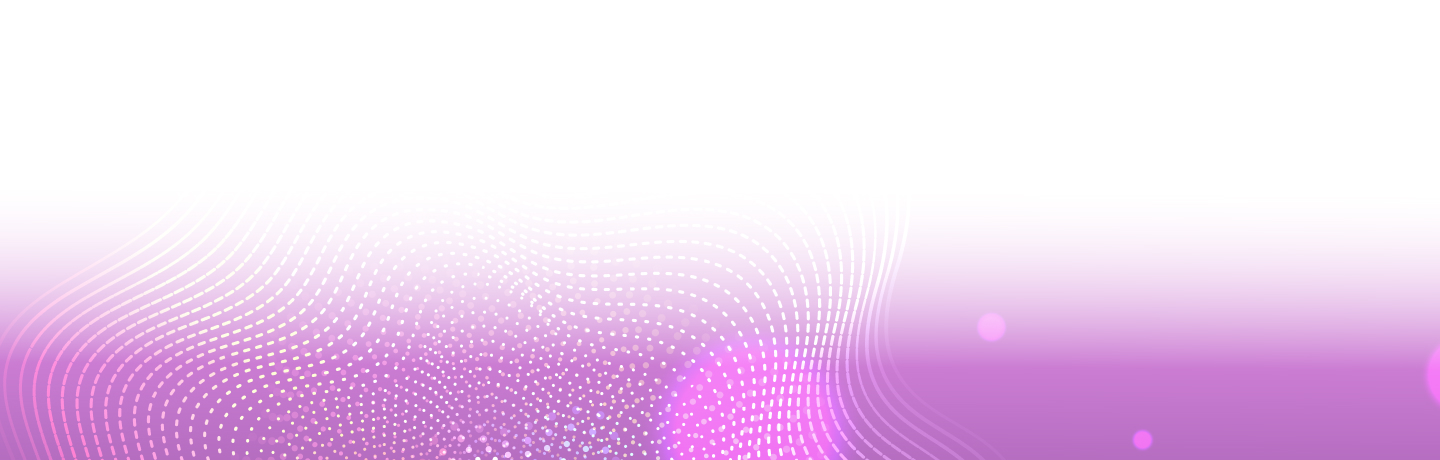