Science cluster

Summary
PRIVAGAMS creates a cutting-edge platform for generating privacy-preserving simulated data using Generative Adversarial Networks (GANs). It ensures high-quality, realistic datasets while protecting sensitive information, with applications in clinical, tabular, and imaging data. The project also focuses on sanitising machine learning models, through advanced techniques, such as model distillation and watermarking, enabling secure research across various RIs.
Challenge
Open Science project, Open Science Service, Cross-domain/Cross-RI
Ensuring privacy while maintaining data utility is a growing concern, especially in sensitive fields like healthcare, where data includes personal medical histories and diagnostic images. Current anonymisation techniques struggle to preserve critical relationships within data, such as links between diagnoses with corresponding imaging data. Additionally, machine learning models can inadvertently retain sensitive information, potentially exposing private data during analysis, particularly in federated learning frameworks.
Solution
PRIVAGAMS will create a platform enabling research institutions to produce high-quality simulated data customised for specific needs, thereby increasing data availability while ensuring privacy remains intact. The project will do so by utilising Generative Adversarial Networks (GANs) to create simulated datasets that closely mimic real data without exposing personal information. These simulated datasets retain the statistical and relational properties of the original data, making them highly valuable for research while safeguarding privacy.
The platform supports multiple data types, including clinical, tabular and imaging data. It also introduces privacy-enhancing techniques for machine learning models, such as model distillation and watermarking, reducing the risk of sensitive data exposure.
Scientific Impact
The innovations of PRIVAGAMS will significantly enhance research capabilities in fields requiring access to sensitive data, such as healthcare and genomics. By allowing the generation of privacy-preserving yet realistic datasets, the platform enables institutions to share data more freely without compromising privacy. Its model sanitisation techniques secure machine learning models against data leakage. With initial integration into BBMRI-ERIC and plans to expand to EuroBioImaging, ELIXIR and EUCAIM, the platform enhances FAIR data management and privacy practices for sensitive data across RIs.
Principal investigator
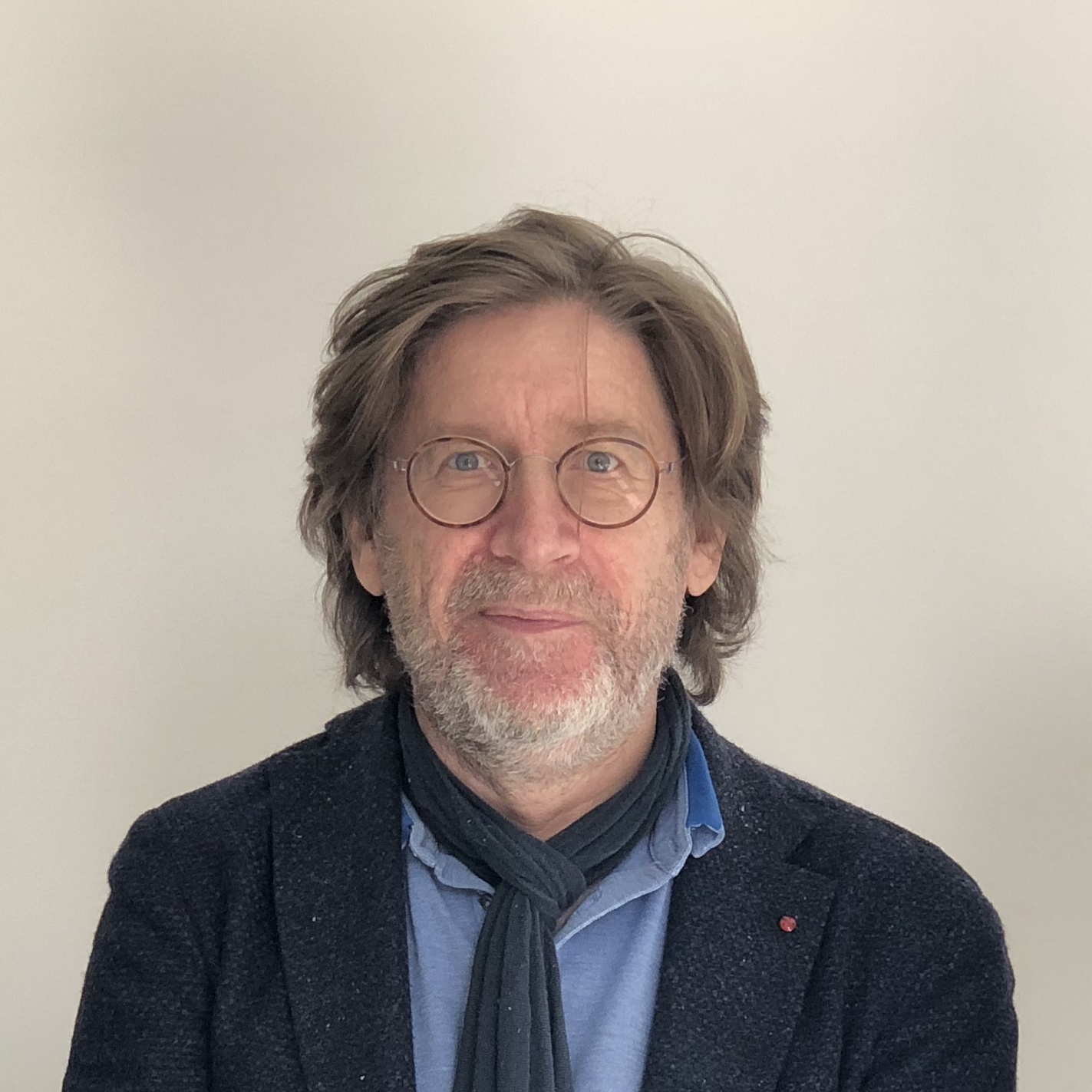
Heimo Müller, PhD, is a mathematician who pursued his studies in Graz and Vienna, culminating in a thesis on data space semantics. He began his career at JOANNEUM RESEARCH in Graz, where he specialised in computer graphics and image processing. After completing a Marie-Curie fellowship at the Free University of Amsterdam, he returned to Graz to focus on Information Design and Information Visualization. He currently heads a research group at the Institute of Pathology, while also working part-time for BBMRI-ERIC, the European Research Infrastructure on Biobanking. Currently, his research interests encompass visual computing, information design, digital pathology, and the explainability of AI in the medical field.
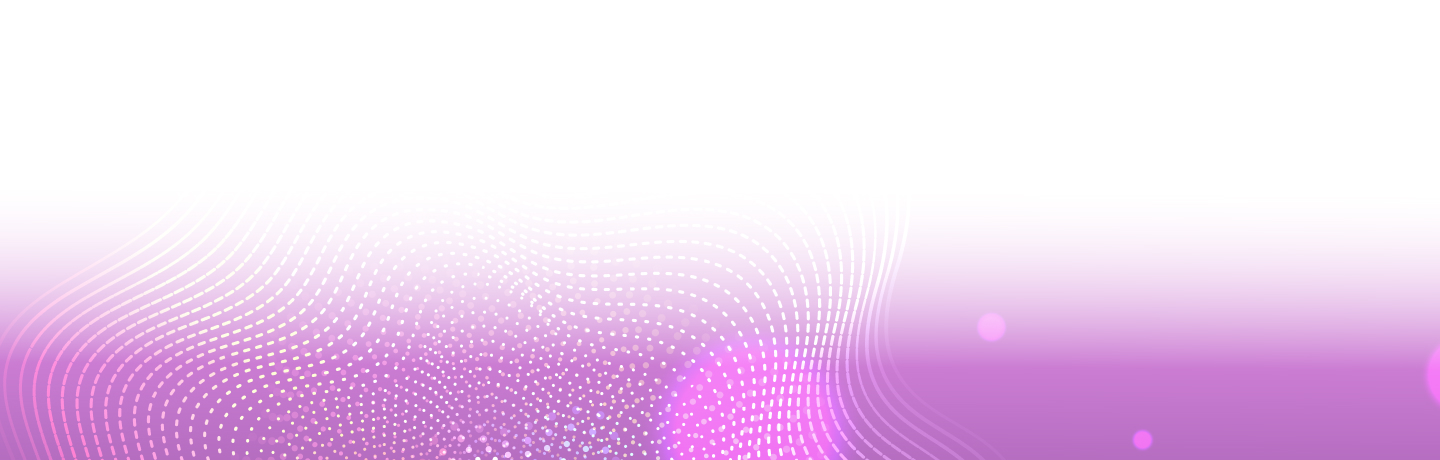